.
Case Study:
Demand Forecasting / International Drink & Brewing Company
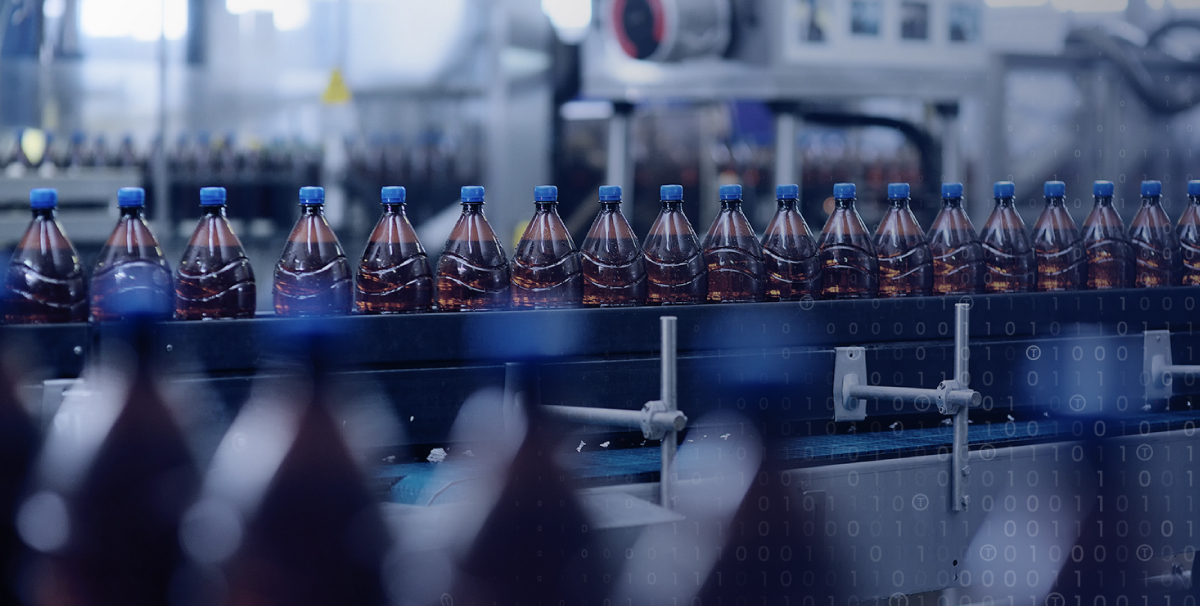
Producing Accurate Forecasts from Massive, Complex Internal & External Data
Global beverage company derives accurate forecasts from 2000+ parameters spanning multiple geographies
Challenge:
Accurately forecast production requirements for 6 international markets using 2,000 internal and external parameters
No company operates in isolation and is therefore subject to market variations at some level, be it because of raw material price variations or more local constraints on customers. As a consequence, demand forecasting accuracy is most often enhanced by the use of data external to a company: in addition to sales data and information on customer behavior, commodity prices (e.g. oil or copper prices), exchange rates, consumer price indices, or even local temperature variations affect demand levels.
However, which parameters are influencial and at what level is often unknown and difficult to uncover, especially in fast moving markets. This is compounded by large amount of data that are usually available within an industry, from historical variations in raw materials, experts projections, consumer price indices, to temperature and humidity variations, and more. As a consequence, it is likely that a large number of models can provide seamingly good predictions but only a few models are robust, simple, do not overfit the data, and produce reliable forecasts.
Solution:
Monte-Carlo randomized process with survival selection combined with Teranalytics Forecast.AI engine
1. First step: Data centralization. Automatically gather and centralize exogenous parameters and combine them with internal data.
2. Second step: Straight time series forecasting. All independent parameters that are not known up to the forecasting horizon are estimated using. Forecast.AI, Teranalytics advanced engine for time series projections.
3. Third step: Automated randomized selection. Teranalytics back-end engine randomly selects independent variables and produces thousands of models that are evaluated for adequacy and simplicity. Selection metrics include accuracy but also sensitivity, specificity, area under the Receiver Operating Characteristic curve, and more.
4. Fourth step: Final model selection. Teranalytics proprietary post-process analysis extracts the most impactful and most robust independent variables to produce a final model that is robust and reliable.
Results:
Forecast accuracy exceeds 90% for 1 and 3 year horizons while dynamically adapting to shifting market data
- Identified top business drivers that could be controlled: out of 2,000 possible external parameters provided, the process ended up producing a final model with only 6 driving parameters. In addition to providing robust and accurate forecasts, this allowed the company to revise their strategy and be better aligned with the market.
- Significantly reduced forecasting resources required: the forecasting process integrated and processed millions of data point in minutes, allowing the company to make the forecasting exercice an integral part of their weekly operation, as opposed to being a one-off exercice dur to the high manpower required.
- Increased investment decision confidence: the forecast accuracy (confirmed with historical data) gave confidence to the order/supply department to create a most just-in-time operation.
Client Feedback:
“We are really happy with this project, and I look forward to using the results at our upcoming Leadership Team All Hands meeting and for all of our subsequent planning.”
– Global Innovations Director –